g and its origins: in favor of network psychometrics of intelligence
Someone sent me this email:
Hello Emil I was wondering if you knew about any similar papers to this one: https://www.sciencedirect.com/science/article/abs/pii/S0160289608001591 Also, what are your thoughts on mutualism (which I assume you think is false)? Does mutualism being wrong disprove gxe theories of heritability? I know it disproves the Flynn model
How about these two? There's probably many more of these if one searches.
Haworth, C. M., Kovas, Y., Harlaar, N., Hayiou‐Thomas, M. E., Petrill, S. A., Dale, P. S., & Plomin, R. (2009). Generalist genes and learning disabilities: A multivariate genetic analysis of low performance in reading, mathematics, language and general cognitive ability in a sample of 8000 12‐year‐old twins. Journal of Child Psychology and Psychiatry, 50(10), 1318-1325.
Panizzon, M. S., Vuoksimaa, E., Spoon, K. M., Jacobson, K. C., Lyons, M. J., Franz, C. E., ... & Kremen, W. S. (2014). Genetic and environmental influences on general cognitive ability: Is g a valid latent construct?. Intelligence, 43, 65-76.
I don't care much about these various models of g factor. I consider the matter empirically undetermined at present. Personally, I think g is a useful index of some brain meta-property. This is basically just following what Arthur Jensen noted on occasion. Since the brain is a neural network, whatever g exactly is, it is related to the network models. Thus, I am happy to welcome more network psychometrics and don't consider these a challenge to g in any important sense. The challenge for these network models will be to explain typical findings in research, such as the inability of training efforts to improve general intelligence despite increases in specific skills. Some recent work on this:
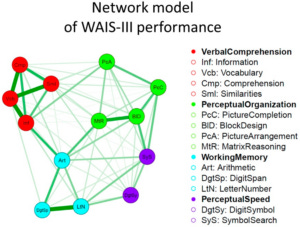
Van Der Maas, H. L., Kan, K. J., Marsman, M., & Stevenson, C. E. (2017). Network models for cognitive development and intelligence. Journal of Intelligence, 5(2), 16.
Golino, H. F., & Demetriou, A. (2017). Estimating the dimensionality of intelligence like data using Exploratory Graph Analysis. Intelligence, 62, 54-70.
Shillcock, R., Bolenz, F., Basak, S., Morgan, A., & Fincham, O. (2018). Understanding greater male variability in general intelligence: The role of hemispheric independence and lateralization. PsyArxiv. 2018.
Yampolskiy, R. V. (2019). Unexplainability and Incomprehensibility of Artificial Intelligence. arXiv preprint arXiv:1907.03869.
Ultimately, this work needs to be merged with the related work on artificial intelligence. The human brain is a supremely complicated neural network, and it's going to be impossible to understand for us. One needs orders of magnitude more complex neural networks to understand a given neural network. Only super-human levels of intelligence can really understand the human neural network. What we can do is collect a lot of detailed data, and then train computers to make predictions for us, and based on these, we can get some useful summary of the underlying model. I consider the various efforts in the neuroscience of intelligence to be actions towards this goal, even though our efforts are really basic at the moment. I follow this field only from a distance as I lack the time to learn to use neurodata, and also these datasets are still mostly hidden away (e.g. UK Biobank, Human Connectome). If researchers wanted rapid progress, they would pool their various private datasets, publish it with some prediction challenge on Kaggle with a decent reward to attract some good talent from the machine learning community. ISIR could spearhead such a challenge, and indeed should. But everybody knows psychologists aren't serious people, so I am not holding my breath.
Edited to add. I should note that the above research of networks is based on cognitive data, whereas where we need to go is network models of neurodata. However, since the cognitive data is a kind of crude mirror of the neurodata, I think whatever advances we make based on 'training on' this will be transferable to the neurodata in time to come. There are also some neuroscience papers using network models. Something like this theory paper is what I have in mind.