Personality correlates of breadth vs. depth of research scholarship
Also posted on the Project Polymath blog. An interesting study has been published:
Thomas S. Bateman, and Andrew M. Hess. Different personal propensities among scientists relate to deeper vs. broader knowledge contributions. PNAS 2015 ; published ahead of print March 2, 2015, www.pnas.org/cgi/doi/10.1073/pnas.1421286112
This is relevant to the study of polymathy, which of course involves making broader contributions to academic areas. The authors' own abstract is actually not very good, so here is mine: They sent a personality questionnaire to two random sample of scientists (diabetes researchers). This field was chosen because it is large and old, thus providing researchers with lots of researchers to analyze. They sent out a couple of thousand of these questionnaires and received received 748 and 478 useful answers. They then hired some other company to provide information regarding the researchers. To measure depth vs. breadth, they used the keywords associated with the articles. More different keywords means more breadth. They used this information as well as other measures and their personality measures in four regression models:
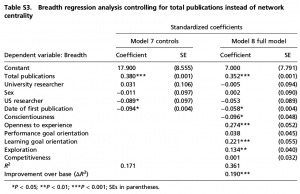
The difference between the sets of regression models is the use of total publications vs. centrality as a control. These variables also correlate .52, so it not surprisingly made little difference.
They also report the full correlation matrix:
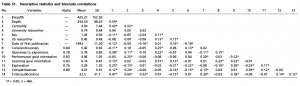
Of note in the results: Their measures of depth and breadth correlated strongly (.59), so this makes things more difficult. Preferably, one would want a single dimension to measure these along, not two highly positively correlated dimensions. The authors claimed to do this, but didn't:
The two dependent variables, depth and breadth, were correlated positively (r = 0.59), and therefore we analyzed them separately (in each case, controlling for the other) rather than using the same predictive model. Discriminant validity is sup- ported by roughly 65% of variance unshared. At the same time, sharing 35% variance renders the statistical tests somewhat conservative, making the many significant and distinguishing relationships particularly noteworthy.
Openness (5 factor model) correlated positively with both depth and breadth, perhaps just because these are themselves correlated. Thus it seems preferable to control for the other depth/breadth measure when modeling. In any case, O seems to be related to creative output in these data. Conscientiousness had negligible betas, perhaps because they control for centrality/total publications thru which the effect of C is likely to be mediated. They apparently did not use the other scales of the FFM inventory, or at least give the impression they didn't. Maybe they did and didn't report because near-zero results (reporting bias).
Their four other personality variables correlated in the expected directions. Exploration and learning goal orientation with breadth and performance goal orientation and competitiveness with depth.
Since the correlation matrix is published, one can do path and factor analysis on the data, but cannot run more regression models without case-level data (ETA 2018: actually, one can do this using path models based on the matrix). Perhaps the authors will supply it (they generally won't).
The reporting on results in the main article is lacking. They report test-statistics without sample sizes and proper (d or r, or RR or something) effect sizes, a big no-no:
Study 1. In a simple test of scientists’ appraisals of deep, specialized studies vs. broader studies that span multiple domains, we created brief hypothetical descriptions of two studies (Fig. 1; see details in Supporting Information). Counterbalancing the sequence of the descriptions in a sample separate from our primary (Study 2) sample, we found that these scientists considered the broader study to be riskier (means = 4.61 vs. 3.15; t = 12.94, P < 0.001), a less significant opportunity (5.17 vs. 5.83; t = 6.13, P < 0.001), and of lower potential importance (5.35 vs. 5.72; t = 3.47, P < 0.001). They reported being less likely to pursue the broader project (on a 100% probability scale, 59.9 vs. 73.5; t = 14.45, P < 0.001). Forced to choose, 64% chose the deep project and 33% (t = 30.12, P < 0.001) chose the broad project (3% were missing). These results support the assumptions underlying our Study 2 predictions, that the perceived risk/return trade-off generally favors choosing depth over breadth.
Since they don't mention the SDs, one cannot calculate r or d from their data I think. Unless one can get it from the t-values (not sure). One can of course calculate relative ratios using their mean values, but I'm not sure this would be a meaningful statistic.
Their model fitting comparison is pretty bad, since they only tried their preferred model vs. an implausible straw man model:
Study 2. We conducted confirmatory factor analysis to assess the adequacy of the measurement component of the proposed model and to evaluate the model relative to alternative models (21). A six-factor model, in which items measuring our six self-reported dispositional variables loaded on separate correlated factors, had a significant χ 2 test [χ 2 (175) = 615.09, P < 0.001], and exhibited good fit [comparative fit index (CFI) = 0.90, root mean square error of approximation (RMSEA) = 0.07]. Moreover, the six-factor model’s standardized loadings were strong and significant, ranging from 0.50 to 0.93 (all P < 0.01). We compared the hypothesized measurement model to a one-factor model (22) in which all of the items loaded on a common factor [χ 2 (202) = 1315.5, P < 0.001, CFI = 0.72, RMSEA = 0.17] and found that the hypothesized six-factor model fit the data better than the one-factor model [χ 2 (27) = 700.41, P < 0.001].
Not quite sure how this was done. Too little information given. Did they use item-level modeling or? It sort of sounds like it. Since the data isn't given, one cannot confirm this, or do other item-level modeling. For instance, if I were to analyze it, I would probably have the items of their competitiveness and performance scales load on a common latent factor (r=.39), as well as the items from the exploration and learning scales on their latent factor, maybe try with openness too (r's .23, .30, .17).
Of other notes in their correlations: Openness is correlated with being in academia vs. non-academia (r=.22), so there is some selection going on not just with general intelligence there.