Testing colorism using genomic data without phenotypic data
Normally, testing colorism or other causal models of why human racial traits have nonzero relationships to socioeconomic outcomes requires that one has the following data:
Measure of racial ancestry
Measures of racial appearance
Measures of socioeconomic outcomes such as income or educational attainment
Path model wise, one can think of it this way:
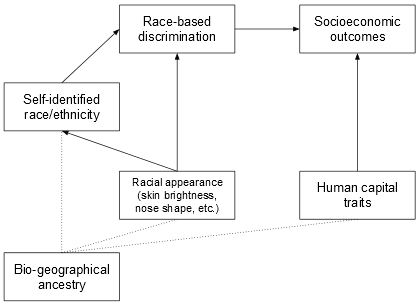
Discrimination models involve the "Race-based discrimination" node, while the familial (genetic or shared environmental) involve the human capital traits route. Thus, a strong test of the colorism model vs. familial models is simply to check whether the racial phenotype x S outcomes link is still nonzero after one controls for the racial ancestry (bio-geographical being the currently popular euphemism). Familial models says it should be zero, race discrimination model says it should be nonzero. Which is right?
Lots of datasets with the required three variables exist, but I have not found any public dataset yet. It may exist and if you know one, I'd like to hear from you. Note that it must be fairly large because the racial appearance x S outcome correlations are often in the area of .10. Thus, the dataset must be able to distinguish clearly between a .10 and a .00 correlation. The standard error of a correlation is calculated by:
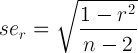
(equation given here, for details see this book, page 42 in the third ed., section 2.8; book is on libgen)
Thus, we algebraically re-arrange this to have n on the left side and get:
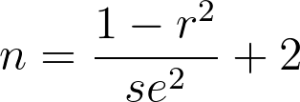
Thus, if we plug in our desired value of se, e.g. .02 and a correlation of .10 and .00, we get the sample sizes required, which are 2477 and 2502. This is definitely within the realm of possibility of typical medical studies. Furthermore, one could combine several datasets to one larger dataset (integrative data analysis, the superior method of meta-analysis).
Genomics meets sociology
Still, suppose we cannot get such a dataset for whatever reason. Can do we something else? Yes. We can use genomic datasets with no phenotype data at all. This may be much easier to get. The only requirement is that we have SNP-level data for the participants and they must belong to a racially admixed population with substantial variation in S outcomes. If we have that, we can estimate the racial appearance traits using GWAS results (such as this GWAS for facial features, and this GWAS for various racial phenotypes). Then we do the same for S outcomes using GWAS for e.g. educational attainment (e.g. Okbay et al 2016). Finally, we estimate the racial ancestry itself using standard admixture methods.
With these, it's a simple matter of checking if racial appearance has any effect beyond that of racial ancestry itself using multiple regression. For the less statistically inclined, what this does is ask the following questions:
When looking at genetic data only, do persons with more genes for brighter skin have more genes for higher income than one would expect based on their overall level of European ancestry?
When looking at genetic data only, do persons with more genes for wider noses have fewer genes for higher education than one would expect based on their overall level of African ancestry?
and so on for other combinations of ancestry and racial appearance traits
It is clear that a racial discrimination model predicts that these questions should be yes, whereas the familial models predict that the answer should be no. Thus, it allows for strong inference.
One caveat of this is that it relies on the heritable part of the variation in these traits. Both racial ancestry and phenotypes have heritabilities near 100% and also have fairly simple genetic architectures, so they are (/will soon be) easy to predict from genomic data. Socioeconomic outcomes are not as heritable (e.g. educational attainment perhaps 40% but seems to be increasing) and have very complex genetic architectures. The result is that our correlations will be very small. The correlation that matters is the correlation between our genomic prediction scores and the real scores. The latest GWAS found that these polygenic scores explained 3.2% of the variance, which is a correlation of .18. So, if the correlation between the racial appearance and the S outcome is .10, this becomes about .018 in our data. Thus, we need a sample size that can distinguish between a correlation of .00 and .018, a tiny difference. How large would such a dataset need to be? Suppose we want a standard error of .0025, n = 160k. Large indeed, but considering that we need no phenotype data at all, this data can come from just about any source as long as it contains data on the same population e.g. Mexicans or African Americans.
This is assuming the current GWAS results. Supposing that we soon reach the breaking point Hsu is positing and perhaps start using better methodology as well, then perhaps we can predict most of the heritable variation in educational attainment. Suppose we could predict 75% of it, i.e. 75% of 40%, so 30%, which corresponds to a correlation of .55 or about about 3x the current (.18). With that, the expected genomic correlations would be .055 and .000 and we could get away with a much larger standard error, perhaps .01. This would require a sample size of only 10k -- already well within reach.